Machine Diagnosis
April 28, 2020essay,
Ramon Amaro signals traces of political arithmetic thought in government responses to the COVID-19 pandemic. Amaro goes into the wider implications of human quantification and data consolidation and asserts that COVID-19 analysis tools, vaccine research, and detection devices risk leaving open future gaps in data privacy, while also centralizing the patient data into government forecasting efforts. We must consider what impact data consolidation might have on future, even non health related, surveillance programmes. It must be asked whether the risks involved outweigh the immediacy of crisis; and if so, what traces of political and economic speculation based on our intimate medical data might be left behind. This essay is part of the publication and research project of Open! about sense of touch in the digital age.
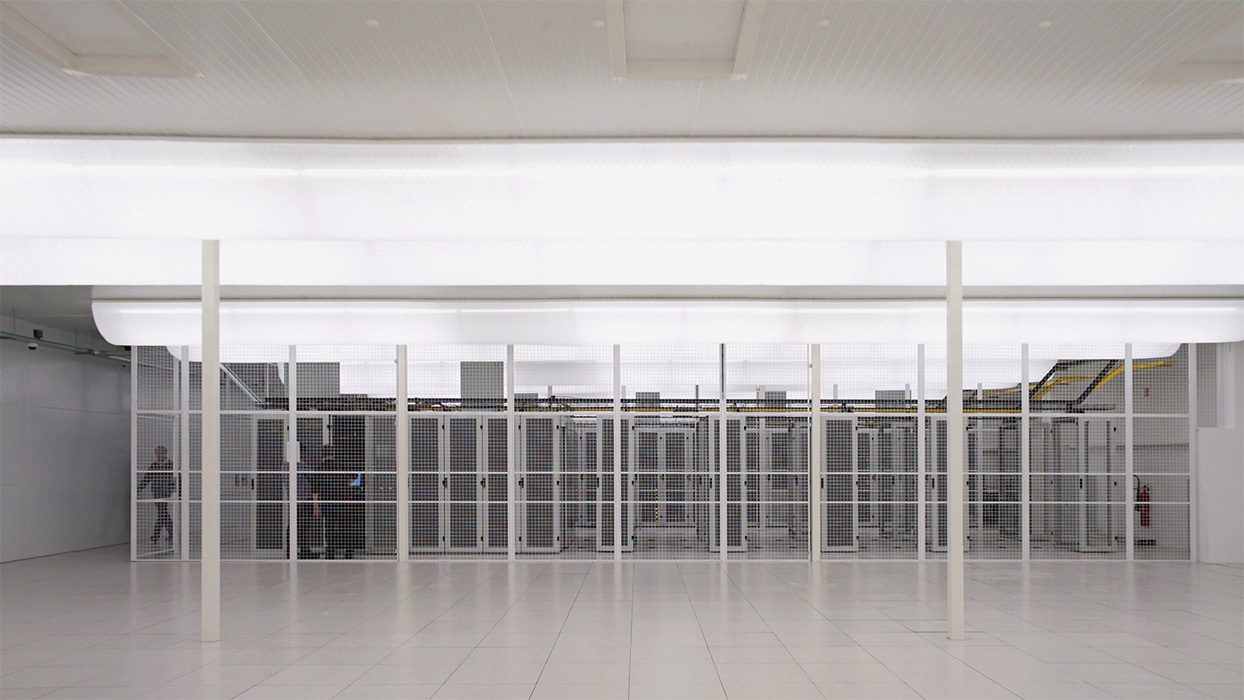
In early 2020, Damo Academy, the research unit of Chinese multinational technology company Alibaba, announced the release of an AI system for the diagnosis of the COVID-19 (coronavirus).1 The system is said to detect COVID-19 in patient computed tomography (CT) scans with 96 per cent accuracy over ordinary viral pneumonia. The algorithm was trained with sample data from over 5,000 confirmed coronavirus cases, as well as data on treatment guidelines and recently published health studies. The algorithm was first released to medical professionals at Qiboshan Hospital in the Zhengzhou Province of China, with plans for adoption across additional provinces. In addition to great accuracy, the new algorithm is said to complete the virus detection process in 20 seconds or under, compared to the five to 15 minutes it would take a doctor to analyse more than 300 images in a CT scan.2
Alibaba is among many tech companies, from AlphaGo’s Deepmind to surveillance company BlueDot, that are rapidly developing new machine learning and artificial intelligence (AI) algorithms for COVID-19 patient diagnosis. These algorithms are in addition to the creation of so-called ‘smart’ devices, such as the Kinsa ThermoScan 5 Ear smart thermometer and app, which combine user temperature readings with other user-generated data to provide real-time detection; and – as founder Inder Singh says – ‘real-time response’.3 The ThermoScan device for COVID-19 is powered by a repurposed algorithm that was originally developed to predict and detect the spread of the traditional flu virus. The ThermoScan algorithm is not the only one that has been repurposed for use in the COVID-19 pandemic. Damo used a previous machine-learning algorithm to develop a public health service app that could answer inquiries about the SARS-CoV-2 coronavirus and its spread. Deepmind has announced that they are releasing a new version of the AlphaFold system to help detect protein structures that can promote research around COVID-19. Toronto-based health surveillance company BlueDot Surveillance is compiling data from various online sources, including airline flight information, to make predictions on where the coronavirus might spread next. SenseTime’s facial recognition algorithms are being used to scan the faces of people wearing masks to help promote contactless identification of suspected carriers. The SenseTime temperature detection algorithm has already been deployed in underground train stations, schools, and other public spaces in and around Beijing, Shanghai, and Shenzhen.4
Due to the time-sensitivity of the COVID-19 pandemic, the scientific community has in response built on decades of research, organized around the sharing of open access data, as well computationally predicted data structures. However, traditional peer-review and verification processes are being circumvented in order to contribute to larger global scientific research. ‘Normally we’d wait to publish this work until it has been peer-reviewed for an academic journal’, states the DeepMind blog.5 The protein structure predictions in their latest version of AlphaFold ‘have not been experimentally verified’. Yet, they ‘hope they may contribute to the scientific community’s interrogation of how the virus functions, and serve as a hypothesis generation platform for future experimental work in developing therapeutics’.
Public Health vs. Public Privacy
While data and machine learning have been readily enlisted to accelerate COVID-19 detection and response, there are limitations to taking a predominantly algorithmic approach to public health. Research suggests that humans may be slower at detecting the virus, but faster than algorithms at recognizing the significance of its circulation.6 This is because there are inconsistencies in how different early disease detection organizations report medical data, according to Nita Madhav, CEO of San Francisco-based disease-monitoring firm Metabiota. These inconsistencies can lessen the accuracy of an algorithm’s performance, particularly those that use natural language processing to scan online texts, social media posts, and medical case studies. Madhav suggests that low data quality can potentially confuse algorithms and skew outbreak prediction. As a result, humans are included in the process to ensure that the data is reviewed properly. His warning is in response to Google’s Flu Trends service, which is perhaps one of the most significant cases of biased hypothesis generation. In 2008, Google launched Flu Trends to detect flu outbreaks by looking for patterns in web search data, such as queries about flu symptoms. The service was heavily criticized for overestimating the number of occurrences of the virus. Flu Trends was discontinued as result, and its technology was given over to non-profit organizations to help them build their own models.7 However, it is unknown (at least to the public) how much of these data were kept by Google for other non-therapeutics uses.
At risk in the increased demand for vital statistics are issues of privacy, most significantly how the consolidation of personal data might result in increased centralization of surveillant technologies. Data consolidation is data generated from many disparate sources and in various different formats. These data are collected from any available source and not limited to any specific time, location, or purpose. Data consolidation offers important benefits in the race for COVID-19 detection and prevention, making it easier to access, manipulate, and analyze a greater set of data. In one sense, data consolidation adds value by achieving enormous time savings, decreased costs, and higher algorithmic efficiency. On the other hand, data consolidation is an irreversible process. Once personal data is consolidated into new algorithmic training sets, they lose their original context and become part of new software scripting. Scripts can, as in the examples stated above, be repurposed for surveillant algorithms that extend beyond their original context – in this case, the tracking of the COVID-19 virus. In efforts to solve one contextual machine-learning problem, the champions of any new consolidated data pipeline (for instance, Google DeepMind, Damo Academy, or even government security agencies) might claim proprietary ownership over the personal data well beyond the immediate COVID-19 pandemic. Even if data are released to the public as open source, ownership of these data sets are most often transferred under existing claims of propriety.
Privacy advocates Latanya Sweeney and Ji Su Yoo argue that although personal medical data is considered highly sensitive information in many countries, these data are vulnerable to breaches in privacy and security.8 Despite best efforts to protect sensitive data through anonymization and encryption, many medical records still rely on a unique identifier for patients. These identifiers are often the same identifiers that are used across other official government and administrative records, such as national insurance numbers, employment documents, credit card and banking applications, taxation, and other administrative processes. Sweeney and Su Yoo have shown that although encrypted, these identifiers are presumed to be anonymous when the patient’s name and address are removed, and can be easily de-anonymized. The researchers argue that personal medical data is still at risk for de-anonymization, especially if the data has been transferred in ownership across several companies and agencies – each with their own security protocols and quality control processes. For example, Sweeney and Su Yoo conducted two de-anonymization experiments on 23,163 encrypted Resident Registration Numbers (RNN) from prescription data of South Koreans. The researchers demonstrated that embedded within the prescription data was demographic information that could be traced back to other publicly known numerical patterns such as credit card numbers, phone numbers, email addresses, passwords, and related RNNs. Sweeney and Su Yoo cite several cases of major data breaches at private South Korean companies. For instance, a July 2011 security breach at SK Communications, which owns a popular social networking platform, left many South Koreans at risk of theft and fraud. The researchers demonstrated how the exposed personal data could be used to further de-anonymise user medical records and other patient data. Sweeney and Su Yoo argue that these concerns extend beyond national borders. For instance, in February 2013, a lawsuit was filed by 1,200 physicians and 900 private individuals against IMS Health, a large multinational corporation headquartered in the United States, which collects medical data and RNNs of millions of South Koreans. According to the researchers, IMS Health claimed that they did not violate patient privacy because the data was reasonably encrypted. Sweeney’s and Su Yoo’s ultimate aim was to provide a better understanding of risks to privacy, at a time when many countries become more data-driven, and either restructure or consider adopting national identification systems.
On the Terms of Life, Death, and Data
Some note that the de-anonymization of data is a necessary risk in the global race to solve the COVID-19 crisis. After all, as journalist Ram Sagar posits, twenty-first century problems require a twenty-first century approach.9 Rightly so, the need for time-sensitive efforts to lessen the impact and de-escalate the current crisis cannot be understated. Despite global urgency, any effort at mitigation of the crisis demands an unprecedented cooperation between humans and machines, which will – even unintentionally – result in the exponential growth of consolidated data sets in the public and private spheres. However, as the immediate threat of COVID-19 passes, many key questions will remain. Among them are concern over the future use of the COVID-19 data outside of the therapeutic domain. Where will this new population data be stored? To whom will it be made available? And, ultimately, who or what will maintain expertise and ownership over any publicly derived vital data captured during the current crisis?
While the consolidation of vital data, public health analysis, and surveillance might appear to be a twenty-first century problem, contemporary data-driven approaches to societal understanding emerge from a longer history of mathematical application to social investigation. By social investigation, I refer to the process by which human and non-human phenomena are abstracted into fragments of data by means of mathematical theory and analysis. Traces of the sudden application of mathematics to societal phenomena can be seen as early as the seventeenth century, when the aggregation of detailed data on populations became common practice among amateur statisticians in the United Kingdom as well as continental Europe. Although data aggregation and statistical exercises did not originate in Europe, or in the seventeenth century, the century marked an important shift in the collective use of data throughout European countries. Prior to the seventeenth century, there were very few mathematical methods to explain the world in which we live. However, as new statistical methods began to emerge, the world – as well as the people within it – came into view as an exercise in pattern recognition.
The Emergence of a New Science
Much of the statistical activities during the seventeenth century were championed by amateur statisticians who had a personal interest in social observation.10 It was believed that statistics and these new sources of data could offer clues to help statisticians better understand the world around them. Amateurs formalized their observations into small ‘life tables’ (also known as ‘mortality’ or ‘actuarial tables’), which contained semi-anonymous data on individual characteristics such as age, place of birth, trade, ‘fighting men’, the number of burials, and in many cases causes of death such as chronic disease. Markers such as these were used to make quantitative judgement by seeking numerical regularities within the data, which could be attributed to what was thought to be a causal relationship between human social patterns and the laws of nature. Amateur hobby coincided with more a general public fascination with scientific advances in the areas of astronomy, geometry, optics, music, and mechanics. Among these discoveries was a new statistical understanding of human life as that which can be approximated with the causal certainty of natural phenomena such as the consistent rising and falling of the sun or the growth of spring harvest.
As data aggregation increased in popularity, statistics became the preferred method for empirically driven knowledge production. Life tables expanded into a myriad of subclassifications, such as the rate of urban over rural death, the proportion of tradesmen afflicted by a certain chronic disease, or the excess of male over female births.11 Data and statistics became powerful tools for human classification and the serious study of human-impacted phenomena such the growth of domestic commerce and international trade, the reproduction of labour forces, and the impact of epidemics such as the plague on the political economy. For instance, in his 1662 publication Natural and Political Observations on the Bills of Mortality, English demographer John Graunt collated data from sources such as the Bills of Mortality, an official record of weekly causes of death in London. Graunt’s aim was to create a statistical model that could predict the spread of the bubonic plague, including data on public burials that were found in the public domain and more proprietary government surveys on death records from local villages throughout the United Kingdom.
With nature and mathematics in tow, Graunt’s cause to connect issues of birth, mortality, labour, economics, and health became attractive tools for the realization of political governance, and more specifically population control. Although mass data aggregation originated in amateur practice for private use, the practice gained prominence as a wider political strategy. Shichiro Matsukawa notes that Graunt dedicated his work in Natural and Political Observations on the Bills of Mortality to Lord J. Roberts, minister of state under King Charles II who was an avid patron of statistics as the ‘new science’.12 Matsukawa writes:
It is undeniable that Graunt’s study, motivated by his personal interest, was too crude in construction to inquire into the true nature of the subject observed and too obscure in basic thinking to clarify the socio-economic relationship. This notwithstanding, his discovery of regularities in social phenomena was of such significance at that time, especially because the regularities were numerically demonstrated with indisputable evidence, that Graunt, shop-keeper as he was, was given the membership of the Royal Society with the King himself as recommender.13
Although Graunt’s numerical models were never fully realized, his methods did inspired new belief in the statistical study of governance based on population and societal data.14 A prominent example is political arithmetic, introduced by English politician and physician Sir William Petty around 1671 or 1672. Political arithmetician Charles Davenport describes political arithmetic as ‘the art of reasoning by figures, upon things relating to government’.15 The definition political arithmetic summarizes the extensive use of numerical analysis to all matters related to the state, from the survey of households and family demographics to the calculation of revenues, live stock, taxation, poverty relief, military power, and so forth.
Statistics as a Manner of Standing
For Graunt, Petty, Davenport, and other political arithmeticians, statistics was foremost political. The measure of reason ‘by figures’ effectively transferred amateur interests in data into the heart of Parliament. The etymology of the word statistics is illustrative of the important role numerical analysis has played in the political imaginary. The word statistics derives from the Latin status, and shares its root with the word state, meaning ‘manner of standing, position, condition’.16 For political statisticians, statistical analysis supported growing political and economic sentiments around the role of governance as the guardians of stability. Statistics provided a sense of security against otherwise unpredictable population, subsequently labour, and economic behaviour.
As such, statistics provided a practical utility for the understanding of human and non-human populations, which was thought to provide a more disciplined and efficient means of economic and political administration, as well as a more granular investigations into public life, which could now be surveilled in ‘Number, Weight, or Measure’.17 More so, statistics was thought to provide a much more comprehensive evaluation of institutional structures than humans alone, freeing governance from ‘prejudice, credulity, and superstition’, as John Arbuthnot explains.18
Matsukawa argues that Graunt’s efforts as a merchant and self-taught statistician were primarily motivated by personal interest rather than an intention to solve social problems.19 Graunt’s intention was to develop a universal measure for trade. Matsukawa posits that as commercial arithmetic (later political arithmetic), or the building of predictive data models using of mathematics, had progressed in England into the first decades of the seventeenth century, statistical methods were primarily derived for purposes outside of academic study. Statistical methods and the study of mathematics were instead seen as cultivators of a social economy that could be universalized into a series of causal factors that might explain a potential loss in productivity and manufacture. By quantifying regularities in population phenomena, Graunt attempted to demonstrate that human patterns were inherently economic, and could therefore be numerically regulated by the laws of nature, or more explicitly the laws of God. Thus, any human or economic regularity could be imagined as the Divine Truth of nature that could be, as result, anticipated with a degree of certainty.
Alain Desrosières posts that, while Graunt (a tradesman) and Petty (a physician, mathematician, and member of the Parliament of England) saw potential in statistical as a new kind of God-sanctioned science, they more so positioned themselves as experts in all matters of population phenomena ‘with a precise field of competence who suggests techniques to those in power while trying to convince them that, in order to realize their intentions, they must first go through them’.20 Desrosières suggests that the distinction between research and the promotion of expertise is a matter of a particular course of action, in which any knowledges produced in the former are wilfully consolidated into the latter as a display of individual power.
Life as Statistics …
Although the use of political arithmetic is believed to have declined after the eighteenth century, researchers like Julian Hobbit and Shichiro Matsukawa argue that ideals surrounding political arithmetic have had far-reaching influence in the widespread adoption of numerical observation.21 Not only had population surveys risen in popularity within governance, but they were published widely in private and commercial sectors. In fact, ‘every [Western] state’, as Ian Hacking explains, had become ‘statistical in its own way’.22 This is no better illustrated than in the significant increase in population surveys in public census records, and the present assignment of national identification numbers to individuals that are either born or reside in certain territories, nations, or states. As with contemporary practice, individual national numbers are universal identifiers that organize a number of human and non-human phenomenal, such as taxation, insurance, credit and consumer, as well as patient and medical records.
Desrosières argues that keeping records on baptisms, marriages, burials, and other characteristics and life phenomena is linked to concerns with determining individual identity as that intended for economic and administrative means.23 Donna Jones posits that life, understood under these terms, ‘has now becomes nothing more interesting than a specific kind of information in an information age’ that fulfils the fantasy of empirical provenance.24 Jones argues that despite early attempts at revealing the mysteries of life (or death) by means of empiricism, the actual condition of being human is incomprehensible. Life is enlisted as the subject of technological experimentations and discovery, yet remains distant from the lived experiences of those observed and quantified. Statistics serves as a medium for the transmission of a simulated reality conveyed as natural law, whereas in actual ‘actions of a people are mediated by the culture that they themselves have created, they exhibit a heightened form of freedom from natural mechanical causality that a purposive organism exhibits in its life activities’.25 Within this regime, life is abstracted into empirical method and recast as that which has value only in as much the fragments of existence can be collected, traced, and measured.
… and, the traces left behind
Although this brief exegesis is not meant to cover the vast history of statistical analysis, it aims to illustrate a potential opening into the wider implications of human quantification and data consolidation. Given the historicity of the numerical analysis on population health, including the abstraction of health crisis like widespread disease into the value of labour and political economy, additional concerns might be raised as to the longer-term impact of contemporary data consolidation. While data and numerical analysis remain significant indicators of COVID-19 spread and detection, it is useful to consider how present data-driven analysis might set in motion new trajectories of society investigation, particularly as more commercial and government-sponsored agencies shift computational resources towards the consolidation of personal information. This exercise is most readily seen in widespread tracking of mobile phone data to monitor the trajectory of COVID-19 infection, or the frantic aggregation of health-related web searches that are being leveraged as valuable public health tools.26 These examples are in addition to the repurposing of existing data sets and machine learning algorithms into COVID-19 analysis tools, vaccine research, and detection devices. Data sets comprised of personal medical and health information risk additional exposure to breaches in security and other malign uses. Thus, it is necessary to keep in full view what has already gained precedent in historical approaches to public health, management, and well-being.
Despite these claims it is imperative that we consider what literal traces of life might behind after COVID-19. It would be foolish to speculate on any certain outcome of what could very well be one of the most comprehensive consolidations of personal data in human history. It remains to be seen if any approach to social investigation during this crisis will materialize into more concentrated modes of governance, or if individual and collective lives as they are might reinforce existing notions of political and economic value beyond the crisis at hand. This is not to undermine the need for more advanced solutions to local and global health crisis, particularly during unprecedented expansion of life-threatening pathogens. We carry a responsibility to actively promote the value of individual and collective life, which may require the amplification and expansion of local and systematic efforts. Within our new, more concentrated assemblage of data, disease, and human abstraction are traces of historical methods of social investigation that attempt to reimagine life and death as phenomena that are motivated by the empirical imaginary. This imaginary has in many instances, as seen in the example of political arithmetic, produced alternative sets of human values organized around the centralization of power and the exercise of social falsification. While throughout the seventeenth century new numerical resources were limited to early statistical theory, we are armed today with an array of powerful machine learning and deep data mining algorithms that can rapidly produce societal insight.
While it is difficult to establish a clear causal link between political arithmetic and contemporary numerical analysis conducted in the search for COVID-19 solutions, there are traces of political arithmetic thought in government responses to the pandemic. Governmental responses to COVID-19 in the UK, in particular – where there is an unprecedented consolidation of social data – are reminiscent of a numerically led strategy to pandemic recovery. For instance, the UK Department of Health and Social Care (DHSC), which is responsible for government policy on health and adult social care matters in England, and overseer of the English National Health Service (NHS), has issued a five pillar testing strategy to understanding trends and risks to public health so as to control and prevent the spread of COVID-19. The pillars include: 1) the scaling up of NHS swab testing for those in medical need; 2) Mass swab testing for critical key workers; 3) Mass antibody testing to help determine immunity; 4) Surveillance testing to learn more about the disease; and 5) Spearheading a Diagnostic National Effort to build a mass-testing capacity. Concerning pillar 4, specifically, the Department of Health and Social Care states that:
Robust population surveillance programmes are essential to understand the rate of infection, and how the virus is spreading across the country. They help us to assess the impact of measures taken so far to contain the virus, to inform current and future actions, and to develop new tests and treatments.27
Part of the ‘robust’ population surveillance programmes are mandates that general practitioners share confidential coronavirus patient information with the UK Government.28 GPs are asked to adhere to data protection regulations ‘within reason’ to ‘support the secretary of state’s response’ to COVID-19.29 Government-approved IT contractors TPP (which runs a health service app) and EMIS (a clinical support system), are also asked to share consenting patients’ data with the UK Biobank project, a national and international health research repository established in cooperation between the UK government and various health research foundations.30 Although GPs are asked to keep records of all the data processed under the mandates, it is unclear where this data will be stored, how this data will be used post-virus, or under what conditions these data might be subject to the privacy agreements of external organizations.
Swift movements to consolidate data by agencies such as DHSC risk leaving open future gaps in data privacy, while also centralizing the patient data into government forecasting efforts. Nonetheless, it is premature to speculate on how these new insights might impact the future of social living, economic or political life. Given the brief genealogy of a key moment in numerical analysis and the contemporary response to the COVID-19 pandemic, we must consider, however, what impact data consolidation might have on future, even non health related, surveillance programmes. It must be asked whether the risks involved outweigh the immediacy of crisis; and if so, what traces of political and economic speculation based on our intimate medical data might be left behind? Although reasonable efforts to secure our information seem to remain a priority, history has shown that what might appear to be steps towards the immediacy of crisis mitigation may lead to the propagation of far more widespread than the contemporary biological virus.
1. Sun Henan, ‘Alibaba says AI can identify coronavirus infections with 96% accuracy’, Nikki Asian Review, 19 February 2020, asia.nikkei.com
2. As of 19 February 2020. See ibid.
3. Thor Benson, ‘Going Viral: Machine Learning Might Help US Predict Where The Coronavirus Will Spread To Next’, Inverse, 9 March 2020, inverse.com.
4. Sam Sagar, ‘11 Ways AI Is Fighting Coronavirus Outbreak’, Analytics India Magazine, 11 March 2020, analyticsindiamag.com.
5. ‘Computational predictions of protein structures associated with COVID-19’, DeepMind, deepmind.com.
6. Matt O’Brien and Christina Larson. ‘Can AI flag disease outbreaks faster than humans? Not quite’, Associated Press 20 February 2020, apnews.com.
7. Sasikiran Kandula and Jeffrey Shaman, ‘Reappraising the utility of Google Flu Trends’, PLOS Computational Biology 2 August 2019.
8. LaTanya Sweeney and Ji Su Yoo, ‘De-anonymizing South Korean Resident Registration Numbers Shared in Prescription Data’, Technology Science, 29 September 2015.
9. Sagar, ‘11 Ways AI Is Fighting Coronavirus Outbreak’.
10. See Ian Hacking, The Taming of Chance (Cambridge, UK; New York: Cambridge University Press, 1990).
11. Hacking, The Taming of Chance.
12. Shichiro Matsukawa, ‘Origin and Significance of Political Arithmetic,’ The Annals of the Hitotsubashi Academy 6, no. 1 (October 1955): 53–79.
13. Matsukawa, ‘Origin and Significance of Political Arithmetic’, 58.
14. See Hacking, The Taming of Chance.
15. Julian Hoppit, ‘Political arithmetic in eighteenth-century England’, Economic History Review 49, no. 3 (August 1996): 516–40.
16. See Michael Wood, Making Sense of Statistics: A Non-Mathematical Approach (Basingstoke: Palgrave, 2004).
17. See Hoppit, ‘Political arithmetic in eighteenth-century England’.
18. Ibid., 520.
19. See Matsukawa, ‘Origin and Significance of Political Arithmetic’.
20. Alain Desrosière, The Politics of Large Numbers: A History of Statistical Reasoning (Cambridge, MA: Harvard University Press, 2011), 24.
21. See Hoppit, ‘Political arithmetic in eighteenth-century England’.
22. Hacking, The Taming of Chance, 16.23.
23. See Desrosières, The Politics of Large Numbers: A History of Statistical Reasoning.
24. See Donna V. Jones, The Racial Discourses of Life Philosophy: Négritude, Vitalism, and Modernity (New York; Chichester: Columbia University Press, 2012).
25. See Ibid.
26. Leo Kelion, ‘Coronavirus: Apple and Google team up to contact trace COVID-19’, BBC News, 10 April 2020, bbc.co.uk.
27. gov.uk.
28. Constanta Pearce, ‘GPs told to share Covid-19 patient information with Government’, Pulse, 7 April 2020, pulsetoday.co.uk.
29. ‘Coronavirus (COVID-19): notice under reg 3(4) of the Health Service Control of Patient Information Regulations 2002-NHSE, NHSI’, UK Department of Health and Social Care, 23 March 2020, assets.publishing.service.gov.uk.
30. ‘Coronavirus (COVID-19): notice under reg 3(4) of the Health Service Control of Patient Information Regulations 2002-Biobank,’ UK Department of Health and Social Care, March 23, 2020, assets.publishing.service.gov.uk.
Ramon Amaro is a lecturer in the Department of Visual Cultures at Goldsmiths, University of London; visiting tutor in Media Theory at the Royal Academy of Art, The Hague (KABK); and former Research Fellow in Digital Culture at Het Nieuwe Instituut in Rotterdam. His research interests include machine learning, engineering, black ontology, and philosophies of being. Ramon has completed his PhD in Philosophy at Goldsmiths, while holding a Masters degree in Sociological Research from the University of Essex and a BSe in Mechanical Engineering from the University of Michigan, Ann Arbor. He has worked as Assistant Editor for the SAGE open access journal Big Data & Society; quality design engineer for General Motors; and programmes manager for the American Society of Mechanical Engineers (ASME).